The dilemma due to the negative correlation between them
The Bulletin Pharmaceutical Company claims to have developed a new drug by researching existing medications to enhance therapeutic effects. To verify this claim, the Ministry of Food and Drug Safety (MFDS) formulated a hypothesis and conducted hypothesis testing through experiments. In this scenario, should the MFDS be cautious about concluding that the new drug's effect is essentially the same as the existing drug but with better efficacy? Or should they be cautious about concluding that the new drug's efficacy is actually better, but the effects are deemed similar to the existing drug?
In this debate, we will explore fundamental statistical concepts essential for understanding errors in hypothesis testing. Additionally, we will work through examples of Type I and Type II errors, examining situations where minimizing Type I error is crucial and vice versa. If you can read and fully comprehend this text along with the introduced statistical concepts, you would be able to confidently answer the question above.
Hypothesis Testing
Hypothesis testing involves statements made by researchers about a population or its parameters1 based on probabilistic samples. It is a process of assessing the truth of a previously formulated hypothesis using sample data. The null hypothesis (H0) serves as the starting point for the test and assumes that the hypothesis under investigation is true. The alternative hypothesis (H1) presents a statement opposite to the null hypothesis, representing what the researcher aims to prove. The null hypothesis is the actual subject of the test, and the alternative hypothesis is automatically accepted when the null hypothesis is rejected.
Therefore, it is advisable to set hypotheses such that those that are statistically challenging or impossible to test are framed as alternative hypotheses rather than null hypotheses. For example, in solving statistical problems, the null hypothesis should include the equality symbol (=), while the alternative hypothesis should avoid including the equality symbol. If one wants to determine if 'a' is on average greater than 'b', the null hypothesis should not be a>b but rather set a>b in the alternative hypothesis and a≤b in the null hypothesis. Thus, researchers must carefully consider the conditions and context related to the problem to adopt a proposition that can be realistically proven as the null hypothesis.
Setting the null and alternative hypotheses correctly is crucial in hypothesis testing. No matter how accurately the test is conducted, if these hypotheses are incorrectly formulated, the test results may be completely opposite. Applying this to real-world issues, beyond simply focusing on equality symbols, researchers must consider all external factors. Therefore, researchers should meticulously review the statistical problem they are dealing with to accurately formulate the null and alternative hypotheses.
Type I Error and Type II Error
Hypothesis testing is generally aimed at statistically proving that the null hypothesis is not true. However, the decision about which hypothesis is correct is always subject to potential errors, as it relies on the analysis of collected statistical data. Sampling errors always occur, so hypothesis testing based on them inherently carries the risk of errors. Hypothesis testing errors can be classified into Type I and Type II errors.
Type I error occurs when the null hypothesis is true, but it is rejected. The probability of Type I error is denoted as α, and the maximum value of Type I error probability is referred to as the significance level2. Type II error occurs when the null hypothesis is false, but it is not rejected. The probability of Type II error is denoted as β, and 1-β is called the power of the test3. Minimizing both Type I and Type II errors is desirable in hypothesis testing. However, in situations where the sample size is fixed, reducing one type of error tends to increase the other.
As seen in the figure, reducing the size of α by shifting the critical value 4to the right naturally increases the size of β, and vice versa. Therefore, the person conducting the test can control the error level but cannot simultaneously minimize both types of errors. The so-called "hypothesis testing error dilemma" arises from conflicting opinions on whether to reduce Type I error or Type II error. We will explore both perspectives through examples below.
The Case of Type I Error
"Innocent until proven guilty" is the principle of presumption of innocence, stating that the accused or suspect is presumed innocent until proven guilty. This principle, explicitly mentioned in our country's constitution, holds great significance in a democratic state. The presumption of innocence not only serves to prevent wrongful punishment and unjust harm but also plays a crucial role in penetrating the essence of the case and uncovering the truth. Therefore, in the principle of presumption of innocence, the null hypothesis and the alternative hypothesis can be formulated as follows.
Null Hypothesis(H0) |
The defendant is innocent. |
Alternative Hypothesis(H1) |
The defendant is guilty. |
In a court of law, following the principle of the presumption of innocence, the null hypothesis assumes the defendant's innocence, stating, "The defendant is not guilty." In this scenario, the plaintiff and the prosecution, seeking to prove the defendant's guilt, formulate the alternative hypothesis as, "The defendant is guilty." Now, let's explore the possible errors that can occur when testing these hypotheses.
Type I error |
The defendant is convicted when he isthey are actually innocent. |
Type II error |
The defendant is released when he isthey are actually guilty. |
Type I error occurs when the null hypothesis is true, yet it is rejected, leading to the wrongful conviction of the defendant. On the other hand, Type II error occurs when the null hypothesis is false, but it is not rejected, resulting in the failure to recognize the defendant's guilt. In this case, the defendant, despite being a criminal, remains unpunished.
Certainly, in this example, Type II error is also highly consequential. Especially if the perpetrator has a high recidivism rate or has committed a serious crime, it could pose significant problems for the broader community. However, legal professionals emphasize the importance of preventing even one innocent person from being wrongly accused, even if it means letting ten guilty individuals go free. Therefore, in this example, minimizing the occurrence of Type I error is deemed appropriate.
The Case of Type II Error
We have been experiencing the impact of COVID-19 from 2020 until now. I dare say that there is hardly anyone reading this who is unfamiliar with COVID-19. In that case, were you aware that hypothesis testing errors are also utilized in the process of COVID-19 testing? Let us explore this through the following example.
Null Hypothesis(H0) |
The test subject is negative. |
Alternative Hypothesis(H1) |
The test subject is positive. |
As mentioned earlier, the null hypothesis is the hypothesis we consider to be true. In other words, it is the accepted hypothesis when there is no compelling evidence that the alternative hypothesis is true in ordinary circumstances. In the context of COVID-19 testing through rapid antigen tests and self-diagnostic kits, the assumption is that the test subject is negative, given the lack of definite evidence supporting the alternative hypothesis. Next, in the scenario of COVID-19 testing, Type I error and Type II error are defined as follows.
Type I error |
The test result says positive when the test subject is actually negative. |
Type II error |
The test result says negative when the test subject is actually positive. |
Type I error is referred to as a false positive, while Type II error is termed as a false negative. In the case of Type I error, if it occurs, the individual being tested might believe they are COVID-19 positive and choose to undergo additional PCR testing or enter self-isolation. The issues that can be qualitatively and quantitatively measured in this situation include:
1. The inconvenience of having to undergo an unnecessary PCR test.
2. If the individual proceeds directly to self-isolation without further PCR testing, they may be unable to carry out their usual activities for a week.
3. The government may waste resources and incur unnecessary costs by conducting a PCR test that was not required.
Now, considering the consequences of Type II error:
1. The individual may fail to adhere to the necessary self-isolation, leading to numerous instances of COVID-19 transmission in the community.
2. The person, even if personally negative for COVID-19, might be mistaken for having the virus, resulting in not receiving necessary COVID-19 support such as medications or supplies.
3. If the individual continues testing due to suspicion despite the negative result, additional costs may be incurred.
4. From the government's perspective, failure to isolate one individual could lead to substantial losses, including PCR testing costs, wasted manpower, et cetera.
In this example, which do you think results in a greater societal loss, Type I error or Type II error? The answer is Type II error.
Conclusion
Then, lastly let us answer the first question in the introduction. I am pretty sure that you can answer this question by yourself. Now, I will give you the answer. The answer would be Type I error which is MFDS should be cautious about concluding that the new drug's effect is essentially the same as the existing drug but with better efficacy. The reason for this is because if patients believe that the new drug is much better than the original, they will keep trying that drug whose efficacy is actually the same. Then, they might miss the golden time while just relying only on medications that do not work.
Generally, in statistics, Type I error is considered more critical. Therefore, researchers usually fix the probability of Type I error at the minimum level and set the rejection region 5to minimize the probability of Type II error. Despite that, as we saw in the example above, there are clearly cases where reducing Type II errors is more important. The reason for this discrepancy arises from the inherent tension between academic and real-world problems. No matter how challenging a theoretical problem may be, there is a distinct disparity between controlled academic issues and the multitude of external factors involved in real-world problems. Therefore, when formulating hypotheses and conducting tests in reality, it is crucial not to uniformly reduce the possibility of Type I errors but to establish an appropriate rejection region depending on the circumstances. As it is the best way to make the optimal decision in the face of dilemmas.
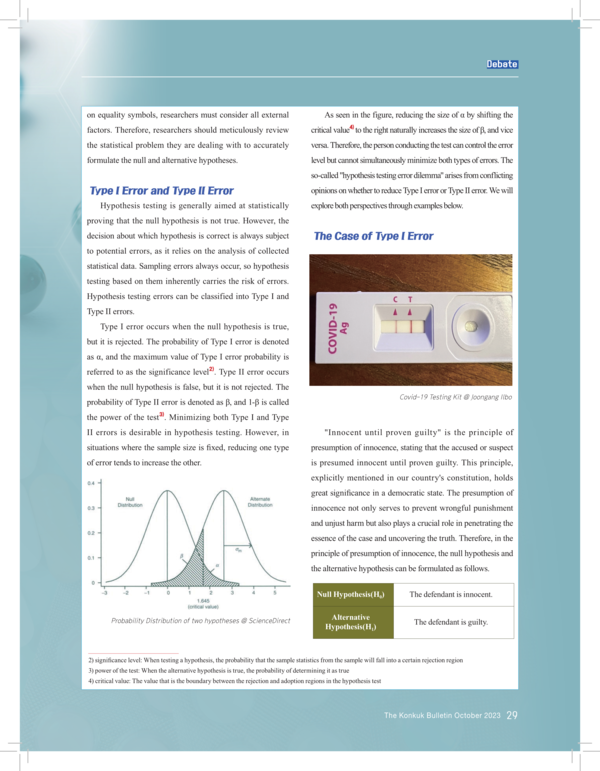
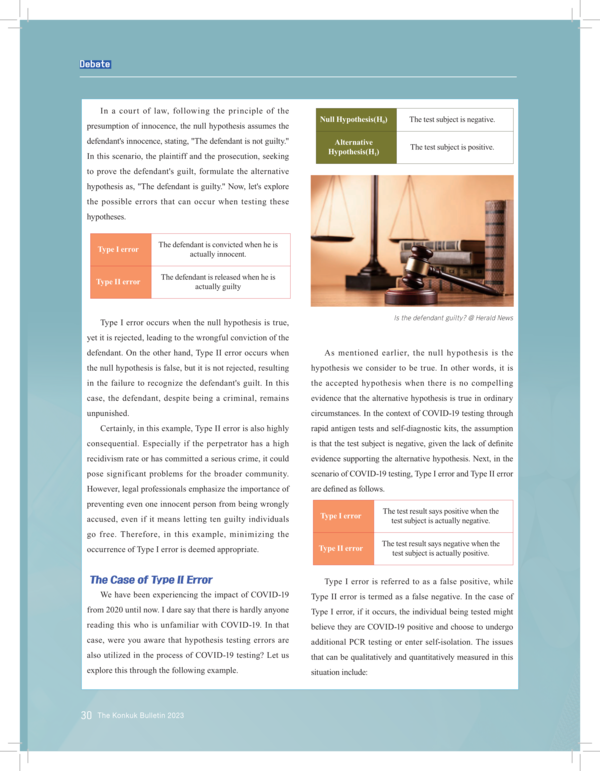
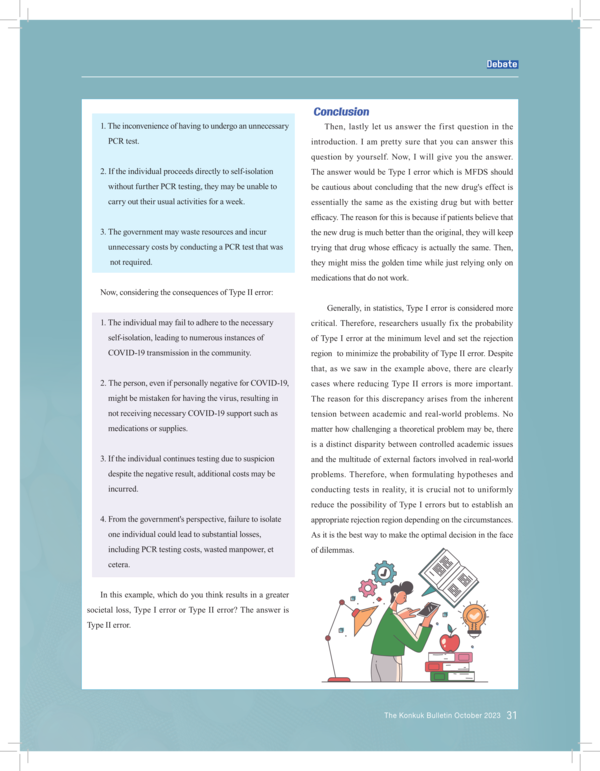